Monica T. Dayao
Computational Biology PhD Candidate @ CMU. Spatial Biology Researcher. CMU-Pitt CompBio.
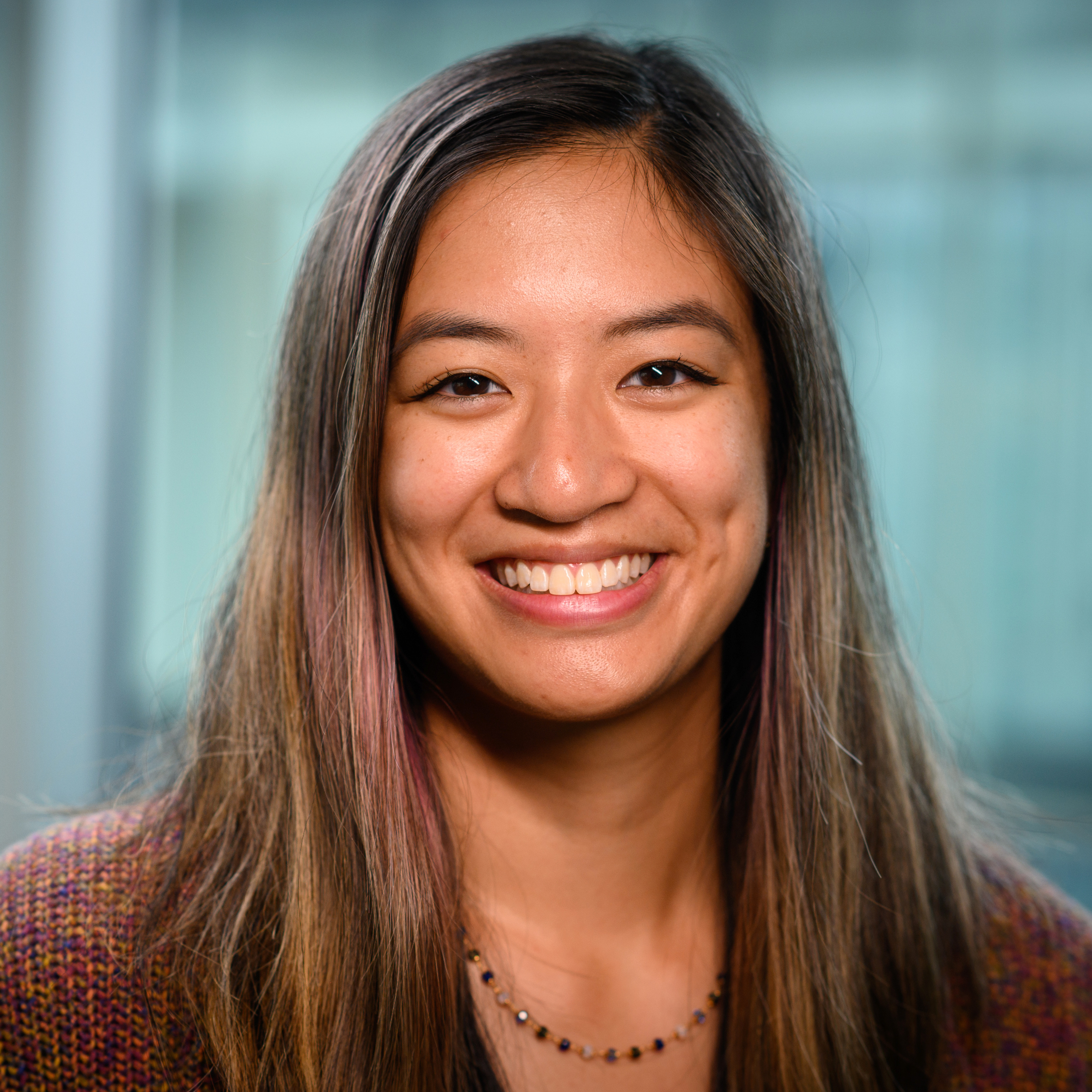
Hi! I am a Ph.D candidate in the Systems Biology Group at Carnegie Mellon University, advised by Prof. Ziv Bar-Joseph. My current research focuses on developing machine learning methods for the analysis of spatial proteomics datasets, as part of the Human BioMolecular Atlas Program (HuBMAP). I also work with collaborators at Enable Medicine and the University of Pittsburgh Medical Center (UPMC).
Research Interests:
- machine learning for genomics and proteomics
- spatial statistics
- deep learning
- computer vision
- survival analysis and prediction
- unsupervised/self-supervised learning
Previously: [CV]
I earned my BA and MEng degrees in Engineering, with specializations in Information Engineering and Bioengineering, from the University of Cambridge. There, I worked with Dr. Timothy O’Leary and collaborated with Prof. Jim Haseloff on deep learning approaches for the segmentation of plant microscopy images.
Misc.
During my free time, I enjoy climbing (find me at Iron City Boulders!), cooking/baking, biking, golfing, and playing volleyball. I own a small van named Mochi, and am (slowly) working on converting it to a campervan! One day I may make some blog posts about the process.
news
Aug 4, 2023 | I received the “Best Oral Presentation” TransMed 2023 Award for my presentation at ISMB/ECCB 2023. Check out the CMU news article. |
---|---|
Jun 30, 2023 | My ISMB/ECCB 2023 proceedings paper has been published in Bioinformatics. Check it out here! |
Apr 11, 2023 | My paper “Deriving spatial features from in situ proteomics imaging to enhance cancer survival analysis” has been accepted to the ISMB/ECCB 2023 proceedings! |
Jan 9, 2023 | I have been awarded an NIH Kirschstein-NRSA F31 award from the National Library of Medicine. This award will fund my stipend, tuition, technical supplies, and conference travel for the remainder of my PhD. |
Dec 12, 2022 | I successfully defended my thesis proposal on “Machine learning methods for the analysis and modeling of highly multiplexed spatial proteomic data” and officially became a PhD Candidate. |